Data Architecture and Data Science: What is the Relationship?
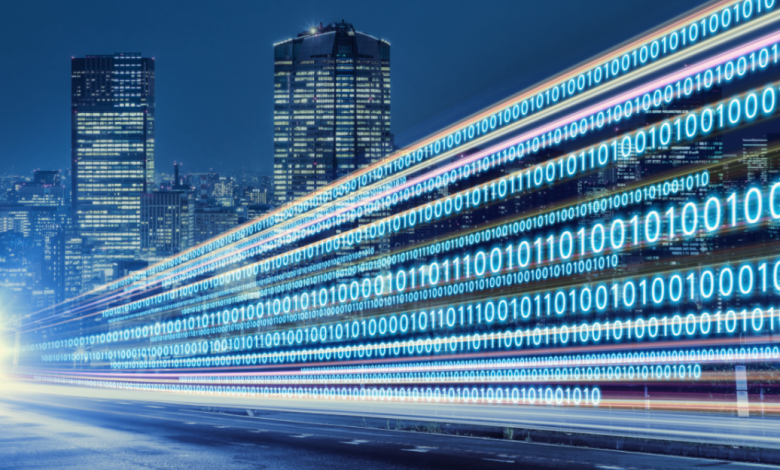
In practise, data science should eventually bring together the greatest practises in information technology, analytics, and business. Data Architecture, on the other hand, allows data scientists to evaluate and exchange data across the company for strategic decision-making. As a result, data scientists will be severely limited in their ability to create and produce data models unless they have a solid Data Architecture in place. This is where Data Architecture and Data Science meet for the first time. (data science course Malaysia)
However, before they can create a model-development and testing environment for business use, both Data Science and Data Architecture specialists must have a thorough understanding of business challenges. An IBM developer investigates Data Science’s architectural approach.
Complementary Roles of the Data Architect and Data Scientist (data science course Malaysia)
Though there are many areas where Data Science and Data Architecture intersect in practise, the data architect is more knowledgeable about hardware technologies, whereas the data scientist is knowledgeable about mathematics, statistics, or software technologies. The data architect converts business needs into technological requirements, establishes data standards and principles, and creates a model-development framework for data scientists to employ. To create models, data scientists use principles from computer science, mathematics, and statistics.
An Enterprise Data Architecture is multi-layered, with the data-source layer often coming first and concluding with the “information delivery layer.” As a result, numerous expertise may be involved in designing the various levels of a complicated Data Architecture, such as the underlying hardware, operating system, data storage, and data warehouse. Modern data architects are typically multi-skilled, with knowledge of data warehouses, relational databases, NoSQL, streaming data flows, containers, serverless, and microservices. Despite the fact that newer technologies emerge on the data-technology scene on a daily basis, technology providers are still waiting for general adoption in enterprises.
The data scientist is unquestionably in charge at the information delivery’s outer layer. This Dzone article on Data Science for Modern Data Architecture describes how predictive analytics is controlled by Data Science.
Data Privacy Act: Data Architecture Requires Secure Data and Model Storage (data science course Malaysia)
Data Architecture now has the added responsibility of providing secure storage facilities for both historic data and constructed models for periodic audit reasons in the post-GDPR world. In this case, Data Architecture plays a larger role in Data Science practise. This is the second point where Data Architecture and Data Science collide.
Unless data-privacy issues are included into the Data Architecture framework, an organization’s data assets will cease to be assets. Data version control will also become a typical part of enterprise Data Architecture in the near future. The Data Privacy Regulation, as exciting as it is for modern data scientists, also heralds a new era of increased compliance for data science practise.
Where Is the Crossroads Between Big Data Architecture and Data Science?
Why Aren’t Big Data Architects in Demand? Software skills alone are insufficient to establish solid big data development architectures (referred to as “infrastructure” in the text), according to Data Scientists. Data scientists are rarely able to manage the complex hardware (environmental) needs of typical big data projects, despite the fact that the hardware technology sector has matured significantly.
Big data projects are frequently deployed on cloud platforms, and big data architects must be familiar with both big data technological frameworks and hardware environments in order to be effective in real-world projects. During the pre-project buy-in sessions, these senior team members are frequently used to persuade clients. Big data architects possess a rare combination of outstanding statistical, programming, and presentation skills, as well as a thorough understanding of hardware environments. Another area where Data Science and traditional Data Architecture (data engineering) collide is Big Data Architecture.
The “Architect” of Data Science Teams is the Data Engineer.
According to an Altexsoft blog post, the Data Engineer’s job description is as follows:
“The purpose of the data engineer in a multidisciplinary team that includes data scientists, BI engineers, and data engineers is primarily to ensure the quality and availability of the data.”
The data engineer makes sure that the data is ready for analysis and that the analytics infrastructure is ready for data scientists to use. The data engineer serves as the “principal architect” of the data environment, preparing it for further study.
Though the data scientist is primarily a “data analyst” and the data engineer is primarily responsible for preparing data pipelines for analysis, there is some overlap between the two roles, particularly in the implementation of machine learning algorithms in the production stage, according to a KD Nugget post describing the explicit differences between data scientists and data engineers.
The Explorer Data Scientist’s Next-Generation Data Architecture (data science course Malaysia)
In the future, “ad hoc or on-demand” data access for “exploratory” Data Science will be possible thanks to on-demand Data Architectures. The data scientist will want to access data “whenever” and from “anywhere” in the exploratory use cases (platform of choice). Citizen data scientists and business analysts will employ techniques derived from “self-service data preparation” and “data virtualization” in next-generation Data Architectures.
Donna Burbank, a leading Data Strategy expert with more than 20 years of experience, gave a presentation titled Emerging Trends in Data Architecture at the DATAVERSITY® Data Architecture Summit, in which she warned that technology was changing so quickly that it would be “challenging to keep up with the latest innovations in Data Architecture.” In addition, she hosts a monthly webinar series on the same subject.
The Framework for a Healthy Data Science Organization
The Healthy Data Science Organization Framework is a set of guiding principles for data scientists to utilise to create and foster a healthy analytics mentality during the data-analysis process. They intended the framework to aid in the development of a better understanding of the organization’s business, data collection, data modelling, and model deployment, as well as overall Data Management practises.
Are you there yet when it comes to creating a Data Strategy? Keep in mind that a real Data Strategy isn’t a pit stop. It’s where you’ll end up.
There’s a good chance that Data Strategy in 2020 will be somewhere in the middle of those two points. The fact that corporate stakeholders are taking a more active role is contributing to the positive attitude.
In 2020, and for the foreseeable future, the link between data strategy and business strategy will get stronger.
More…
Yes, without this link, firms will merely scrape the surface of what they can do with their data in pockets rather of achieving bigger objectives. “The good news is that more individuals are coming to perceive data as critical to the company’s future success, and business leaders are increasingly embracing data,” stated Thomas C. Redman, Ph.D., President of Data Quality Solutions. “They understand that they must play a part and lead the majority of the efforts.”
It’s impossible to overestimate the value of involving additional business stakeholders in Data Strategy. According to Donna Burbank, Managing Director of Global Data Strategy, “as a result of this, firms have developed a strong understanding of their goals around using data, and have come up with some extremely unique approaches to utilise data for commercial success.” “An encouraging sign is that business stakeholders drive the majority of data strategies we support. “In 2020, I see this tendency continuing,” she said.
There are efforts in place to support the mission of including corporate stakeholders. One of these is the Leader’s Data Manifesto. It debuted in 2017 at the Enterprise Data World Conference with the goal of assisting business users in maximising the value of data. Business leaders may read the Manifesto and contact their data counterparts to explore the principles in further depth, or data practitioners could alert them to it.
More..
One of the data executives who helped write the Manifesto, Redman, believes that in the coming year, more business and data team members will have that dialogue. “Every data person must have a thorough understanding of the business unit on which he or she is working,” Redman added.
The Manifesto’s signatories back up the claim about commercial engagement. Leonis Consulting’s founder, Deepak Bhaskar, wrote on the company’s website:
“I’m a firm believer that I realised the data’s worth only when business executives embrace and exploit it for competitive advantage (rather than passing the buck to IT and relying on them).”
Defining the Data Strategy should include the board of directors — or, if it doesn’t, make it a priority to do so this year, according to O’Neal. Indeed, as more groups arise to promote this vision, that tendency may gain pace this year. They found Digital Directors Network, for example, to help senior executives embrace the data storey in their organisations, she explained.
Is a Holistic Data Strategy in the Works?
If you expect it will resolve all of the challenges that come with having a live Data Strategy by 2020, you are wrong. Many firms are still too preoccupied with tactical data management and data silos to devote time to developing an overarching Data Strategy. O’Neal stated:
“Companies continue to struggle with prioritising data issues holistically in order to optimise effort and reduce rework,” she noted. “Because of the numerous issues they face, they may not believe they have the time to elevate the planning process in order to uncover savings and scalability.”
They aren’t looking at data acquisition holistically across the organisation, which is a huge part of the problem. Data scientists, for example, can define what data they need for a given analysis and then go through a tactical data collecting process tailored to their needs, according to O’Neal.
“Do this a dozen — or several dozen — times, and an organization’s data acquisition process can become much more expensive than if it had thought strategically about its data gathering process,” she added.
Burbank observes that business stakeholders, rather than IT, are often the ones who recognise the value of an integrated strategy. According to Burbank, IT personnel typically don’t want to be saddled with extra procedures or engage with a wide spectrum of end users.
Source: data science course malaysia , data science in malaysia